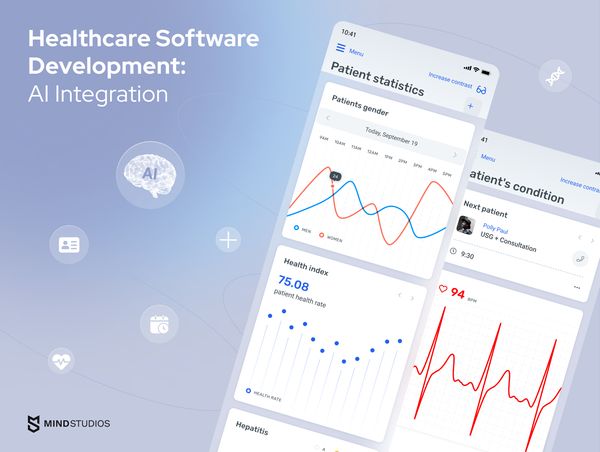
Artificial intelligence has been slowly but steadily working its way into almost every aspect of the healthcare industry. Today, there is no doubt that these technologies are game-changing: AI in healthcare helps discover and test new drugs, spot signs of disease that go unnoticed by humans, and even makes the impossible possible, like enabling remote surgeries.
Moreover, AI is an integral part of streamlining day-to-day healthcare-related tasks and thus making the lives of healthcare professionals a little easier. The question is, how can healthcare organizations start leveraging this power and make AI part of their operations? That’s what this article is all about.
Here, we explore the benefits, use cases, and inspiring examples of medical AI solutions, guide you through the integration process, and share tips on working with artificial intelligence based on our own experience.
Benefits of integrating AI into healthcare software
[Source: Statista]
According to data from Statista, the global healthcare AI market would be worth almost $188 billion by 2030, growing at a CAGR of 37 percent from 2022 to 2030. Let’s get into the benefits of AI in healthcare that have led to such astonishing numbers.
Improved diagnosis and treatment accuracy
One of the biggest advantages of AI-powered algorithms is that they can help quickly and accurately analyze vast amounts of medical data, such as patient records, medical images, and test results in real time. This leads to detecting patterns and anomalies that either take much more time when done by humans or go completely unnoticed without the involvement of AI.
Artificial intelligence helps healthcare professionals (HCPs) apply a proactive approach and provide timely and accurate treatment to their patients, predict and prevent emergencies, and reduce misdiagnosis cases.
Medical professionals are pretty optimistic about the technology. For instance, a 2022 survey by the European Society of Radiology talked to 185 radiologists working with AI-based algorithms to diagnose patients. The majority (75.7%) of them agreed on the general reliability of the algorithms.
Personalized patient care
Ideally, each patient should be treated with consideration of their unique medical history, experience, genetic data, lifestyle, and other information.
AI, in combination with other technologies, can analyze this data in real time. As a result, it enables a personalized approach to care and helps find the best possible diagnosis and treatment solutions for specific patients. This leads to improved patient outcomes and reduces the chances of readmission.
While patients are still cautious about the use of AI by medical professionals, many of them do believe that this technology can solve certain problems in the industry. For instance, the Pew Research Center survey from 2023 showed that 51% of US adults who view ethnic biases in healthcare as a problem believe AI will reduce it. The same research revealed that 65% of US adults want AI to be used in their cancer screening.
Cost savings
While complex decision-making does rely solely on healthcare professionals, AI can relieve them of certain routine repetitive tasks. This includes administrative work like appointment scheduling, patient registration, processing bills, and other paperwork, etc. Automating these operations helps healthcare organizations cut administrative and operational costs.
Moreover, this frees HCPs’ time for patient care. For instance, according to recent research, in Europe, it was estimated that only 50% of a physician’s working time was dedicated to treating patients, and the other 50% was filled with administrative tasks. The implementation of AI in healthcare is forecasted to increase time spent with patients by 20%. This naturally can lead to improved patient satisfaction and therefore increased revenue.
Additionally, AI-powered analytics and predictive modeling solutions can optimize resource allocation in healthcare organizations. For instance, AI algorithms are able to analyze historical and real-time data to optimize staff scheduling and patient flow, improve demand forecasting, and streamline inventory management.
Use cases of artificial intelligence in healthcare software
While there is an abundance of cases artificial intelligence can be used to benefit the industry, most of the AI applications in healthcare can be roughly divided into four categories. These are patient care, diagnostics and medical imaging, research and development, and management. Let’s get into each of these use case groups.
Diagnostics and medical imaging
Artificial intelligence is currently shaping the future of diagnostics and medical imaging, and augmenting human capabilities through advanced algorithms. AI in healthcare software helps HCPs quickly and accurately interpret complex data, and therefore make more efficient medical decisions and reduce medical errors.
Here is a list of typical AI technologies used for diagnostics and medical imaging:
- Machine learning for analyzing large datasets, and thus identifying complex relationships between clinical data, symptoms, and disease outcomes, which helps make predictions and enhance diagnostic decision-making
- Deep learning that utilizes neural networks and helps HCPs with tasks like analyzing medical images, including X-rays, CT scans, and MRIs
- NLP techniques for interpreting human language and analyzing medical records, clinical notes, research papers, and so on to extract relevant information
- Expert systems for simulating human expertise in specific domains through providing HCPs with relevant medical knowledge and clinical guidelines
- Probabilistic reasoning techniques for calculating probabilities and assessing the likelihood of various diagnoses
- AI-powered decision support systems for assisting clinicians with making diagnostic decisions based on real-time data like patient history, symptoms, test results, and medical research
It’s important to note that AI is unlikely to replace humans, and final decisions remain to be the responsibility of medical professionals. However, the use of AI by HCPs does lead to faster and more accurate diagnoses. For instance, in one of our articles, we shared a story of how Hungarian clinics adopted AI systems to help check for signs of breast cancer that might have been overlooked by doctors.
Patient care
When it comes to patient care, AI technologies are primarily used to improve the delivery of healthcare services and care coordination, as well as to enhance patient experiences. They also help healthcare providers embrace a more proactive and patient-centered approach.
Common AI-driven software solutions for patient care include:
- Virtual health assistants powered by AI and NLP that provide patients with personalized healthcare support and guidance. Typically, they can answer questions, provide symptom assessments, offer medication reminders, and connect users with HCPs
- Remote patient monitoring solutions that use ML algorithms and enable HCPs to remotely track patients' vital signs, symptoms, and medication adherence, and intervene when necessary
- Medication management software aimed to assist in medication reconciliation, adherence monitoring, and personalized medication recommendations
- Fall detection and prevention solutions that are used in combination with sensors, AI wearable devices, or camera-based technologies to detect falls or changes in movement patterns. These systems are often adopted by caregivers or emergency services
- Chronic disease management platforms created to support patients with chronic conditions by providing personalized care plans, symptom monitoring, and self-management tools
By leveraging the power of AI technologies, healthcare providers can deliver proactive, patient-centered care, leading to better outcomes and improved overall patient well-being.
Management
Automating administrative and other repetitive tasks is one of the easiest ways for a healthcare organization to start an AI integration journey. These solutions typically are related to operational processes rather than patient care, yet still, they can help save money and increase the quality of services.
Some of the most widely adopted AI-powered software solutions used in healthcare management include:
- Revenue cycle management platforms that help automate and optimize billing, coding, and claims processing, and identify potential revenue leakage
- Supply chain management solutions aimed to optimize inventory levels, reduce costs, and ensure timely availability of critical supplies. In this case, AI algorithms analyze historical data and demand patterns, forecast supply needs, and automate procurement processes
- Fraud detection and prevention services that leverage AI algorithms to analyze patterns and anomalies in claims data, provider behavior, and billing practices, and flag suspicious activities
- Workflow optimization solutions that help automate routine tasks and streamline processes, mainly through Robotic Process Automation (RPA). This technology can cover appointment scheduling, documentation processing, etc.
- Predictive staffing and resource allocation platforms that use AI algorithms to analyze historical patient data, admission rates, and staff scheduling patterns to predict future patient volumes and optimize staffing levels, as well as manage resources efficiently
Research and development
Since AI algorithms can analyze large volumes of medical data, naturally, it’s used not only by healthcare facilities but by researchers as well. AI-powered tools allow scientists to quickly go through massive datasets and identify patterns that accelerate medical discoveries. AI technologies help with the following research-related tasks:
- Data mining and analysis, in which case AI is used to process healthcare data like EHR, genomic data, clinical trials, and scientific literature. This helps researchers understand disease mechanisms better and come up with solutions much faster
- Genomic analysis, where AI and ML algorithms are used to analyze and interpret DNA sequences, predict disease risks, and enhance personalized medicine
- Drug discovery and development, which has become easier thanks to AI and ML algorithms analyzing data on molecular structures, predicting drug-target interactions, and identifying compounds with desired properties
- Clinical trial optimization, in which case AI assists with the optimization of the design and execution of clinical trials. To be more specific, ML algorithms can identify suitable trial participants, predict patient responses, and optimize trial protocols
Using AI for these purposes contributes to the advancement of evidence-based medicine, personalized care, and the overall evolution of healthcare systems.
Examples of AI in healthcare software
It’s much easier to comprehend how this all works with real-life cases, so, for each category, we’ve selected an example of how health tech companies and healthcare organizations are using AI in healthcare software.
Medical imaging: Butterfly Network
As you probably know, ultrasound imaging is a safe, non-invasive, and non-radiative diagnostic tool that allows HCPs to gain images of the inside of the body through sound waves. However, the equipment used for ultrasound is typically very expensive and too large to be used outside healthcare facilities.
That is why a medical company Butterfly Network created the Butterfly iQ, a handheld whole-body imager, which HCPs can carry almost as easily as they carry stethoscopes.
The Butterfly iQ is based on a semiconductor-based ultrasound transducer. The device has thousands of tiny ultrasound sensors, which enables it to make high-resolution real-time images and send them directly to a connected smartphone or tablet.
As for the AI component of the technology, the Butterfly iQ is enhanced with AI algorithms that enhance image quality, optimize settings, and help with image interpretation and detecting anomalies. Additionally, the product leverages cloud computing, which enables data storage, collaboration, and analysis.
Patient care: Wellframe
One of the tasks modern healthcare has to address is patient empowerment, meaning the system needs to enable those under its care to take an active role in managing their health. Wellframe is a service that aims to do just that.
This digital health management platform helps healthcare professionals deliver personalized, interactive, and data-driven care to their patients. To achieve this, its creators combined mobile apps, AI, and care management services.
The Wellframe mobile app for patients is basically a digital health companion with features that enable support and guidance. The functionality includes personalized care plans, medication intake reminders, symptom tracking, educational content, telehealth services, secure messaging with HCPs, and alerting the latter in case of high-risk situations.
Wellframe team also uses AI for healthcare software development. AI algorithms here are used to analyze patient data like health records and create personalized care plans. They also help detect patterns, potential risks, and flaws in treatment, and provide real-time insights and recommendations to patients and HCPs.
Management: Epic
The last example here is well-known to most people in the healthcare industry. Epic is an electronic health records (EHR) system that is widely used for managing patient information and streamlining workflows in healthcare organizations.
Epic’s functionality enables HCPs to easily work with patient registration, documentation, and charting, placing orders for tests and medications, care coordination, and more.
So, what’s the role of AI in healthcare software development by Epic? The platform leverages artificial intelligence for predictive analytics to identify patterns in large amounts of patient data. This way, AI algorithms can predict patient outcomes, detect risks and complications, and enable HCPs to proactively intervene when needed.
Epic also uses natural language processing (NLP) and voice recognition to analyze unstructured data like clinical notes and documentation and extract valuable insights and patterns needed for decision-making. HCPs using Epic are able not only to manage individual patients but support population health management initiatives as well.
Mind Studios team also finds Epic incredibly helpful: in fact, we use it when building HIPAA-compliant solutions for our healthcare projects, including ones that involve AI technology.
Drug discovery: AlphaFold
According to Bloomberg, bringing a new drug to market has typically cost almost $3 billion, with about 90% of experimental medicines failing. AlphaFold, an AI model developed by DeepMind, has significantly sped up and simplified the process by predicting the 3D structure of proteins, which is essential for understating how they are going to interact with the human body.
To put it simply, many diseases are triggered by proteins that behave abnormally. Predicting the 3D structure of proteins helps researchers determine potential drug targets, narrow down molecules that might interact with the proteins, and design drugs to attack diseases.
Here is how it works. Firstly, AlphaFold gets trained with large amounts of data on proteins from available databases, scientific research, and other sources. DeepMind trains the model by using deep learning techniques, specifically neural networks.
The model is then used to predict the protein’s 3D structure and determine how it folds through complex algorithms and computational methods. Its iterative refinement process helps increase the accuracy of the predictions.
Another significant role of AlphaFold in healthcare is that it contributes to creating a comprehensive protein structure database, that can not only boost drug discovery but advance our knowledge of diseases and biology in general.
How to integrate AI solutions into healthcare software
If you are a healthcare organization that already has existing software, it might make more financial sense to invest in the integration of a ready-made AI solution instead of building a new product from scratch. That’s exactly what we want to focus on in this section.
The AI integration process is complex in any field. However, merging AI and healthcare software can be especially challenging due to numerous data privacy and healthcare security AI regulations that need to be taken into account. These six steps will help you better understand how to do it in the most efficient way possible.
Step 1. Define your healthcare organization's needs
The first thing to do when you’ve decided to look into AI integration options is to identify the objectives of this project. What do you plan to achieve with AI solutions? Which processes do you want to automate and enhance? How will it benefit your organization? And what budget can you allocate to the project?
Answering these questions will help you ensure that the idea is valid, and in the end, you will have a list of requirements for the AI solutions themselves and the team that will implement them.
Step 2. Find trustworthy developers to implement the solution
Relevant experience and expertise are of crucial importance when looking for a partner to integrate AI solutions into a healthcare organization’s operations.
Hardware and software service providers need to understand the healthcare industry's regulations, standards, and unique challenges. Therefore, don’t hesitate to evaluate the expertise of your potential partners during technical interviews and contact their previous clients to learn more about their experience and work approach.
Step 3. Choose the right AI solution
There are numerous options for AI tools and technologies. The choice here depends on the type of data you plan to process and its availability, technical requirements of the AI solution, its compliance with regulations, and cost.
Whether you plan to build an artificial intelligence solution from scratch or integrate a ready-to-use one with few adjustments, we suggest making the decision with your experienced AI implementation partner.
Step 4. Plan for integration
The success of your AI project directly depends on the quality and quantity of the data you’re going to train the AI algorithms on. Therefore, one of the crucial steps in preparing for integration involves gathering and analyzing the data.
Depending on the problem the algorithm will address, the data can include medical images, medical transcription, EHRs, data from wearable devices, etc.
It’s also essential to ensure the selected AI solution complies with regulatory requirements and standards like HIPAA and GDPR.
Step 5. Train, test, and deploy the solution
Once the data is ready, you can start training the AI solution and test how accurate the results it generates are. While the key contributors to this process are engineers, make sure you also engage healthcare professionals who will work with the solution in the future since they are the ones who need to validate the effectiveness of the algorithm.
After the AI model has been validated, the team can deploy it, and your in-house team can start integrating it into the organization’s workflow.
Step 6. Monitor and refine your healthcare AI system
The project isn’t over once the solution is deployed: artificial intelligence projects, especially in healthcare, require constant monitoring and improvement.
This process entails gathering feedback from users (in this case — primarily medical professionals and patients), analyzing the impact of the solutions on the healthcare organization’s performance, and refining the AI to ensure desired outcomes.
Challenges and considerations
Despite the positive impact of AI in patient care, it’s still not all rainbows and sunshine yet. Just like most new technologies, this one still has its challenges.
Patient comfort
While the main reason to combine patient care and artificial intelligence is to improve patient satisfaction, we are not there yet. As of 2023, 60% of Americans feel uncomfortable about being treated by healthcare providers relying on AI.
At the same time, 38% of the respondents already believe that utilizing AI in health and medicine will improve patient outcomes. So while there’s no solution to that challenge at the moment, public opinion could potentially improve even more as time goes on.
Patient data protection
Medical data is one of the most sensitive ones. Because of that, it is heavily protected by various regulations such as HIPAA and GDPR. Knowing which projects have to comply with these regulations and which can avoid that is essential for any healthcare app development process.
AI-based tools often need to access lots of patient data to deliver relevant and efficient solutions. At the same time, this could increase the possibility of data breaches and leakages.
Therefore, if you work with AI technology, you have to make data privacy your top priority. This could be achieved by leveraging privacy-enhancing technologies (PETs).
One of the most widespread PET is data masking: another inauthentic although a realistically-looking version of an organization’s data is created to be used for user training, software testing, and other purposes.
Training and education for the healthcare workers
The rapid advancement of AI technologies raises a lot of concerns among various specialists, medical workers included. As AI-based solutions can efficiently perform various tasks, they might potentially replace certain jobs. Therefore, many healthcare professionals are wary about adopting AI.
However, at the moment it seems that AI is here to support the medical workers rather than replace them —and this is unlikely to change soon. So it’s important for tech healthcare professionals to work with the AI technology and to monitor its efficiency to minimize potential risks. Because, after all, artificial intelligence also makes mistakes.
Diagnostic errors
Speaking of mistakes: in the healthcare industry, they could cost not only money but also human lives. That’s why it’s extremely important to prevent them.
As of now, AI can often offer more accurate diagnostics than human specialists (Sanofi’s story supports that), but this isn’t always the case. For instance, hundreds of AI-based tools created to diagnose COVID-19 failed.
In the end, the outcome largely depends on the quality of data used to train the AI solutions. Therefore, it’s up to healthcare organizations to evaluate and verify the data used.
Correct implementation
Not all development teams are familiar with AI and know how to implement it properly. To avoid that, you need to have a clear goal (what you want to achieve with the help of a certain AI technology), high-quality data to train AI on, and a well-defined strategy.
While the goal definition stage is up to you, we at Mind Studios could help you with the other two. We constantly monitor new technology trends in healthcare, AI included, and know how to implement them in an existing product or to design one from scratch for you.
Healthcare industry regulations for AI
In May 2023, Sam Altman, CEO and cofounder of OpenAI, said, "There should be limits on what a deployed model is capable of and what it does." He also recommended lawmakers to pursue new safety requirements for testing tech products before release.
So, why do we mention it in the context of AI technology in healthcare? Well, because even wealthy countries that are actively using artificial intelligence don’t have clear legislative regulations governing the use of AI in the healthcare industry yet. Let’s explore why.
AI regulatory landscape in the UK
As of July 2023, there is no legislation explicitly governing the use of AI in the UK, meaning the use of this technology in healthcare is regulated by general legislation like Data Protection Act 2018 (DPA 2018) and the General Data Protection Regulation (GDPR).
The DPA 2018 and the GDPR are closely linked in the context of the UK. The EU implemented GDPR in 2018, aiming to harmonize data protection laws across member states. And even though the UK departed from the EU, the GDPR continues to apply in the country through the European Union (Withdrawal) Act 2018.
The DPA 2018 was enacted as the primary legislation supplementing the GDPR to ensure data protection and privacy in the UK. The act provides additional details and provisions that complement the GDPR taking into account the UK legal landscape. Both frameworks establish the rules and rights for data protection organizations and individuals must comply with in the UK.
In the context of AI, the DPA 2018 and the GDPR require organizations to:
- lawfully process personal data used in AI systems
- protect data privacy
- ensure transparency and information provision to individuals
- respect rights related to automated decision-making
- adhere to cross-border data transfer requirements
- establish accountability and governance measures
Other UK regulations AI creators in the health tech sector must comply with include the following:
- Regulations by the Medicines and Healthcare products Regulatory Agency (MHRA), the UK regulatory agency that provides certifications aimed to ensure the safety, quality, and effectiveness of medical devices and medicines, including AI-powered devices and software applications used in healthcare.
- National Health Service (NHS) Digital Health Technology Standard, which provides guidance and assessment criteria for digital health technologies, including AI applications. Its main focuses include clinical evidence, data protection, interoperability, and user experience.
- UK Medical Device Regulations 2022 (MDR), which govern medical devices, including AI-powered ones, used in healthcare. The regulations align with the European Union Medical Device Regulation (MDR) and set requirements for the quality, performance, safety, and security of medical devices.
Though there isn’t a regulatory framework that governs AI specifically, in September 2021, the UK government presented the National AI strategy, which contained a plan for the development of AI in the country for the next ten years. Additionally, in July 2022, the UK government published a policy paper establishing a pro-innovation approach to AI regulation.
Currently, the UK’s legislators focus on developing a clear and transparent regulatory framework that would “drive growth while also protecting our safety, security and fundamental values.” For instance, the UK’s National Institute for Health and Care Excellence has been working on a multi-agency advisory service (MAAS) for AI and data-driven technologies, funded by the NHS AI Lab.
The service aims to comprise a partnership between the Care Quality Commission, Health Research Authority, and MHRA and help developers and technology adopters navigate the regulatory landscape. However, it’s too early to tell how successful all this guidance and legislation will be long-term.
EU regulations on AI in healthcare
As we’ve already established, one of the EU’s core legal regulations in AI for healthcare is GDPR, a data protection regulation that sets requirements for the collection, use, storage, and processing of personal data.
While the UK is taking a guidance-based approach, the EU requires a comprehensive and detailed legislative framework since it would be more efficient considering the union has 27 member states.
In April 2021, the European Commission presented a proposal for a regulation laying down harmonized rules on artificial intelligence, also known as the AI Act. The regulation goes hand in hand with the Coordinated Plan on AI. The end goal of the act is to make sure the Europeans can trust the AI solutions they use and contribute to building an ecosystem of AI excellence within the EU.
The AI Act aims to address the risks of specific AI use cases, categorizing them into four levels:
- Unacceptable risk
- High risk
- Limited risk
- Minimal risk
For instance, minimal-risk solutions like secure administrative support automation systems will face no restrictions. Limited-risk solutions will have transparency obligations.
High-risk AI systems will need to go through conformity assessments. Such high-risk systems will likely include robotics assistants used in surgeries, AI systems that provide diagnoses, clinical decision support systems, etc. Such AI-enabled health tech products must have an established risk management system, comply with data governance requirements, and meet several other conditions.
The AI Act is expected to take effect no earlier than in the second half of 2024. The territorial applicability of AI regulations will encompass all member states and, given the Northern Ireland Protocol, Northern Ireland as well.
AI regulations in healthcare for the US
Just like the UK, the US does not have specific comprehensive regulations solely focused on AI in healthcare, though the country’s regulatory bodies do provide guidance on the use of AI in healthcare settings. At the same time, as the EU is working on passing the AI Act, the US has been relatively slow in developing a relevant regulatory framework at the federal level.
As of July 2023, AI technologies in healthcare in the US fall under other existing laws and regulations, specifically those that govern aspects of data privacy, security, and medical device regulations.
Here are the key regulations and guidance sources relevant to AI in healthcare in the US:
- Health Insurance Portability and Accountability Act (HIPAA) sets standards for protecting patients' sensitive health information, also known as protected health information (PHI). Companies and organizations handling PHI, including those using AI, must comply with HIPAA's privacy and security rules to ensure the confidentiality and integrity of patient data.
- Federal Food, Drug, and Cosmetic Act (FD&C Act) regulates medical devices, including certain AI-based software apps used in healthcare. This means AI software used in healthcare can fall under the medical device category and require FDA clearance.
- FDA's Software as a Medical Device (SaMD) Guidance on the regulation of software that functions as a medical device, including AI-based software, outlines principles for determining the risk categorization and regulatory requirements for SaMD.
- National Institute of Standards and Technology (NIST) Frameworks, including the AI Risk Management Framework, provide guidance on assessing and managing risks associated with AI systems.
For companies and organizations developing or managing health tech products powered by AI, it’s essential to constantly monitor the regulatory landscape since artificial intelligence is a rapidly evolving area.
If you are also involved in AI-enabled software development in the US, be sure to follow the latest updates from regulatory bodies like the FDA, FTC, OCR, NIST, and other relevant regulators. Sooner or later, the US will likely develop an industrial strategy for using AI in the industry.
Future of AI & ML in healthcare
The future of AI in medicine isn’t that difficult to predict, at least partially. After all, the technologies we’ve mentioned in this article are yet to be widely adopted and are likely to stay with us, even if in a more evolved form.
The most promising areas for AI solutions to be part of include precision medicine, drug discovery, remote patient treatment, robotic surgery, imaging analysis, and more efficient management of EHR systems. In other words, the future of AI in healthcare holds nothing you haven’t heard of before.
However, there is an aspect of artificial intelligence adoption that many healthcare organizations overlook: our approach to the development and deployment of AI systems in healthcare. Here is what Dr. Gianrico Farrugia, president and CEO of Mayo Clinic, which serves over 1.4 million patients each year, has to say about it:
“The traditional pipeline model relies on a linear series of points, from coming up with new ideas to turning them into stand-alone products which providers and patients then get to use. Instead, a platform approach relies on an ongoing ecosystem of collaboration. We need to bring together providers, medical device companies, health tech startups, patients, and payers to co-create integrated solutions through digital platforms – based on longitudinal patient data and algorithms that continue to learn over time.”
Additionally, Dr. Gianrico Farrugia highlights the importance of protecting the privacy and security of sensitive patient data when adopting AI technology by using a federated data infrastructure.
“Rather than sending data to AI models, we are bringing the AI models to the de-identified data, creating a ‘glass wall’ that gives external collaborators access to results without the data ever leaving the platform.
Mind Studios' tips on the implementation of AI technology in healthcare
The process we’ve described above might seem fairly straightforward. However, implementing AI projects in healthcare comes with certain challenges and doesn’t always go as planned, simply due to the complex nature of both the technology and the industry. Here are a few tips from our team that might help you ensure the success of your idea.
Be aware of AI challenges
While artificial intelligence has the potential to revolutionize the healthcare industry, it’s crucial for anyone adopting the technology to be aware of and address its drawbacks and challenges. While AI models reduce the risk of human error, they have been trained on human-created data and therefore can often be biased.
For instance, imagine a clinic’s historical data shows that a certain racial group is less likely to seek healthcare. Since an AI algorithm is trained on that data, it can be less likely to recommend follow-up treatment for patients from that group, even if the care is needed.
Another major concern healthcare professionals have is the lack of transparency since it’s often unclear how AI algorithms come to certain conclusions, which makes it difficult to spot and address biases.
Surely, these challenges don’t mean you shouldn’t adopt artificial intelligence. Just keep in mind that AI solutions should be used under constant human supervision, especially when treating patients.
Choose a reliable long-term partner
AI projects can take years to develop and integrate: just think of the Sepsis Watch tool, which was first released in 2018 and is still being tested. However, even when the AI model is integrated into a healthcare facility’s workflow, it doesn’t become a silver bullet: such solutions require continuous monitoring, training, and improvement.
Therefore, we suggest choosing a technical partner who is genuinely invested in the success of your project and is willing to work on it over a long period of time, providing maintenance and support services after the solution’s launch.
As a software development company, Mind Studios is focused primarily on long-term cooperation to ensure the project’s final success in the long run. As a result, 70% of our clients rely on our maintenance and support services 3 years after the project launch.
Work side by side with healthcare professionals
AI projects for healthcare organizations cannot be developed without collaborating with the healthcare professionals as primary users of these solutions, at least if you don’t want them to fail.
In addition to solving specific problems, AI tools must have user-friendly and intuitive interfaces and prove efficient in a real healthcare environment. Besides, doctors and nurses are the ones who will test whether the solutions are accurate, bias-free, safe, and responsive to the needs of patients.
Lastly, involving healthcare workers in the development process will up the chances of the solutions being accepted and adopted in the future, which also directly affects the success of the project.
Conclusion
The adoption of AI technology in healthcare made the industry enter a new era, enhancing the experience of delivering and receiving care. While these new solutions help automate operations connected to data analysis, diagnostics, and administrative tasks, healthcare professionals can focus directly on treating their patients and making the latter feel like they are a priority.
For sure, investing in complex AI-powered hardware technology can be too expensive for small healthcare organizations with limited funding. However, there are a lot of affordable ways to leverage AI and even use it to cut costs in the long run by merging healthcare software and AI to streamline healthcare workflows.
With healthcare being one of our focus industries, Mind Studios is happy to help you build an efficient software solution or enhance an existing one with AI technology. Feel free to reach out, and our business development team will assist you with creating a strategy that fits both your requirements and your budget.