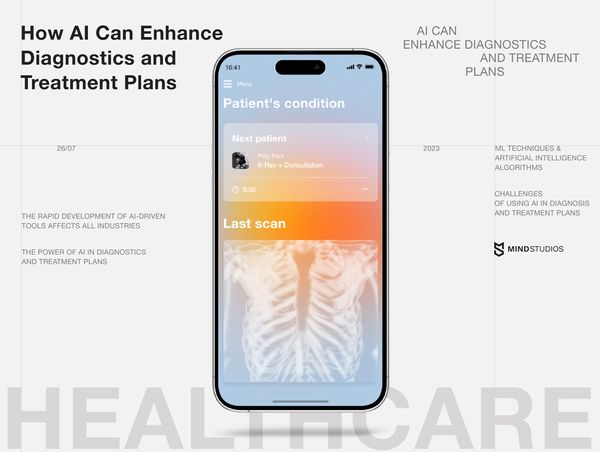
The rapid development of AI-driven tools affects all industries, healthcare included. These days, many healthcare organizations start questioning whether they should implement artificial intelligence in medical diagnosis processes or not. And some companies are already reaping the benefits of such decisions.
Improving the accuracy of medical diagnostics and treatment is indeed a necessity these days. Each year in the US about 371,000 people die and 424,000 sustain permanent disabilities due to the misdiagnosis of diseases and other medical conditions. At the same time, the global AI in Medical Diagnostics market revenue is forecasted to reach $5.5 billion by 2027, growing at a CAGR of 39.9% from 2022 to 2027. The technology seems quite promising.
But is it really promising? Let’s figure this out together. Read further to learn how AI is improving diagnostics, which technologies are there already, and what challenges you might face when deciding to integrate this technology into your products or services.
ML techniques & artificial intelligence algorithms
At the moment, AI in diagnosis and treatment is represented by several main technologies. They are the following:
Boltzmann machine
The Boltzmann machine is a deep-learning algorithm that can analyze complex patterns and relationships in patient data. By doing that, it can diagnose various diseases and predict treatment outcomes, helping doctors make more informed decisions.
K nearest neighbor (KNN)
KNN algorithms are used in medical image analysis. They compare new images to labeled examples, finding similarities. Due to that, they can classify different tissues or detect anomalies, providing valuable insights for diagnosing conditions and assisting radiologists in their work.
Recurrent neural networks (RNN)
RNNs analyze patient data over time to spot patterns and predict future outcomes. They are great for disease progression forecasting, monitoring vital signs, or analyzing patient behavior in time-series data.
Support vector machine (SVM)
Support vector machine (SVM) is another example of AI in medical diagnostics. This algorithm can accurately evaluate and classify medical images, differentiating healthy and disease-stricken tissues. As a result, it can assist in disease classification and predicting how the patient may respond to certain treatments while taking various clinical parameters into account.
Convolutional neural networks (CNN)
CNNs are widely used in medical images. They can quickly detect tumors, segment images, and classify diseases. CNNs analyze and extract features from medical images, helping make a more accurate diagnosis, plan treatments, and monitor different medical conditions.
Long short-term memory (LSTM)
LSTMs are neural networks used to process time-series patient data. These networks spot long-term dependencies and patterns in such data, helping to predict disease progression and patient outcomes, as well as assist in making personalized treatment decisions.
Generative Adversarial Networks (GAN)
GANs can generate synthetic medical images that can be later used for research or training. For instance, they can create realistic simulations to train diagnostic models and improve their efficiency and accuracy. They can also generate synthetic brain scans to augment limited datasets.
The power of AI in diagnostics and treatment plans
Now that you know about these technologies, it’s time to learn about how they can help improve diagnostics and treatment. The most common use cases for artificial intelligence in medical diagnostics are the following:
Early detection of diseases
AI improving disease diagnosis is currently one of the main reasons to implement this technology in healthcare. At the moment, deep learning algorithms already aid in analyzing various types of medical data, spotting patterns that might be difficult for the human eye to detect, and using these patterns to make diagnoses more accurate and quicker.
Last year, Google Health announced its partnership with iCAD, licensing a mammography AI research model. This model was trained on a large dataset of mammograms to identify subtle patterns and early signs of malignancy. As a result, it can support healthcare professionals in making more accurate diagnoses and reducing false positive cases.
Precision medicine
Another major use case of AI in diagnostics is utilizing it to enable personalized medicine. Although at the moment such medicine is considered unaffordable and difficult to access, AI will be able to change that drastically.
By using AI and machine learning to process patient data, healthcare professionals can gain insights into individuals and offer more precise treatments, taking their DNA, their medical history, and their family's medical history into account. This could help build unique treatments and protocols for various diseases, such as Alzheimer’s, diabetes, psoriasis, and others.
For instance, IBM’s Watson for Oncology is an AI-powered system that analyzes vast amounts of medical literature, clinical trials, and patient records to offer personalized treatment options for cancer patients. The system uses the patient's genomic information and medical history to provide evidence-based treatment recommendations, offer more targeted therapies, and therefore increase the chances of successful outcomes.
Medical Imaging Analysis
AI technologies, such as deep learning models and computer vision algorithms, can evaluate medical images (MRIs, X-rays, CT scans, and others), spotting patterns and offering useful suggestions. For instance, in 2021 Tulane University researchers discovered that AI can analyze tissue scans with the accuracy of professional pathologists or even better to detect and diagnose colorectal cancer.
Google Health researchers developed ARDA, an AI system that analyzes retinal images to help clinicians detect diabetic retinopathy and macular edema. The system was trained by a large team of ophthalmologists who used over 100,000 de-identified retinal scans. As a result, it can help with patient treatment in countries that don’t have enough high-quality eye professionals, such as Thailand and India.
Predictive analytics
As AI and ML algorithms are skilled in analyzing large datasets, they also can be used for disease prediction. Such technologies can process data to identify potential risk factors and forecast if a patient is likely to develop a particular disease. Healthcare professionals can use this information to develop preventive measures or early treatment.
In 2017, researchers at the University of Pennsylvania Health System developed a machine learning algorithm that helps predict early signs of sepsis in patients. The tool was trained on the electronic health record (EHR) data from more than 160,000 patients and can monitor hundreds of key variables in real time. This allows clinicians to intervene on time, potentially saving lives and reducing the impact of sepsis on patient health.
Treatment plan optimization
AI can also process vast amounts of patient data and medical literature to optimize treatment plans. CorVista Health platform is a great example of that. It uses AI technology to detect cardiac MRI images and optimize treatment plans for heart disease patients. By examining detailed images of the heart, AI algorithms can identify specific abnormalities, determine the most suitable treatments, and help cardiologists devise personalized care plans for their patients.
How AI can enhance diagnostics and treatment plans
Using AI in healthcare diagnostics has numerous benefits. You can see it for yourself after reading the use cases above. However, the perks of AI usage in this industry don’t end with that.
Here are some of the key benefits of using AI for medical treatment and diagnostics:
Improved accuracy
AI-powered algorithms can process vast amounts of patient data quickly and accurately. This allows them to make more precise and timely conclusions about a patient’s health, reducing the risk of misdiagnoses and medical errors.
As a result, healthcare professionals receive valuable insights that allow them to make well-informed decisions and develop personalized treatment plans. This leads to better patient outcomes and reduces the need for unnecessary tests or treatments, optimizing healthcare resources.
Enhanced decision support
AI in clinical diagnostics can also serve as a powerful decision-support tool for healthcare specialists. Such technologies offer evidence-based treatment recommendations and best practices based on the analysis of patient data, medical literature, and clinical guidelines. Basically, they give clinicians access to the latest medical research and expertise, already processed and well-structured. This, in turn, helps healthcare professionals make more informed decisions regarding patient care.
Access to medical expertise
Unfortunately, not everyone has equal access to high-quality medical expertise these days. Certain regions all over the world suffer from a shortage of specialized healthcare professionals. This, however, can be changed with the help of AI-driven virtual assistance and telemedicine platforms. Such technologies enable remote consultations with medical experts, allowing patients to receive expert opinions and diagnoses from anywhere in the world.
Time and cost savings
AI is also great for automating repetitive and administrative tasks, which, in turn, helps save time for healthcare workers. This technology can be used for managing medical coding, data entry, and appointment scheduling efficiently, giving healthcare staff more time and resources to focus on direct patient care. Additionally, AI can optimize resource allocation, reduce hospital readmissions, and minimize expenditures, helping healthcare organizations to save costs.
Remote patient monitoring
AI-powered remote patient monitoring systems can track the health condition of the patients and help them improve the quality of their life and health. These days, many people use wearable devices such as smart and fitness watches to share their data and receive suggestions from AI algorithms. Although this is mostly done for health and fitness purposes, it’s quite possible that later technologies can use such data to detect changes in health or potential issues. This, in turn, could lead to timely interventions, reduce hospital admissions, and improve overall patient well-being.
Challenges of using AI in diagnosis and treatment plans
While using AI for diagnosis and treatment plans is without a doubt beneficial for many reasons, this technology, being relatively new, also comes with its challenges. The most common of them are the following:
Data quality
Although we keep saying that AI is skilled in processing large volumes of information, the result of this analysis largely depends on the quality of data used. All data, especially the healthcare one, can be sometimes complex, fragmented, and flawed. This, in turn, could lead to unreliable AI-generated outcomes and potentially compromise patient safety.
To solve this challenge, healthcare companies and professionals that train AI algorithms must invest in data management strategies, data cleaning, and validation processes to improve the quality of information used and maximize the effectiveness of AI solutions.
Bias
The same goes for biased data. At the moment, AI algorithms cannot identify if the data you feed them is accurate or not. So if you offer them biased data, they can memorize those biases and view them as facts. This can result in inaccurate diagnoses, poor treatment plans, and even discriminatory statements or suggestions.
To solve this challenge, companies should regularly evaluate AI outputs for bias, and implement strategies to manage or correct any flawed or discriminatory recommendations.
Regulatory issues
This is a general challenge for using AI in healthcare. As patient data is considered a sensitive one, integrating AI solutions into this industry involves navigating complex regulatory frameworks and ensuring compliance with privacy and security regulations, such as the Health Insurance Portability and Accountability Act (HIPAA) in the US or the General Data Protection Regulation (GDPR) in the EU.
To solve this challenge, companies must demonstrate that their AI systems meet the strict standards of data protection and patient privacy. Compliance with regulatory requirements is essential when you’re developing custom healthcare software. This helps build trust with patients and healthcare professionals and to ensure that AI-powered solutions will be used ethically and responsibly.
Cost
At the moment, training, implementing, and maintaining AI systems is quite costly. Although in the long run, it is generally worth the time and funds invested, healthcare organizations should still weigh their options carefully. They should consider the long-term benefits of AI adoption for their particular case to ensure that the result will definitely pay off.
Wrapping up
AI technologies are widely used for enhancing diagnostics and treatment plans these days. They have numerous benefits, such as the ability to quickly process large datasets, spot patterns in patient data, medical imaging, and health records, automate routine tasks for health professionals, and many more. However, implementing AI-driven solutions also has its own challenges, such as data quality and data bias, regulatory issues, and potentially high costs.
At Mind Studios, we understand the challenges that companies may face when integrating artificial intelligence into medical treatment and diagnosis solutions. We are here to help and ready to advise on best practices, problem-solving, and cost optimization. With 10+ years of experience in the software development market and the healthcare application development in particular, we have the knowledge and experience to help you make the right choice.
Don’t hesitate to reach out to us with any questions or assistance needed.