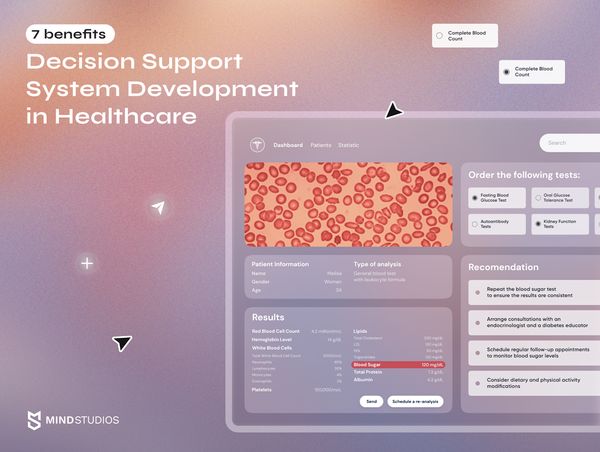
As the demands for healthcare providers grow, turning to decision support system development is not an indulgence but a strategic necessity for healthcare organizations that want to achieve better patient outcomes through innovation and make a difference in their field.
Clinical decision support systems (CDSSs) are specialized applications that utilize data analysis to assist healthcare providers in making informed decisions, thus enhancing patient care. By leveraging knowledge management and data mining techniques, a CDSS provides clinicians with real-time advice, ultimately improving diagnostic accuracy and the quality of patient care.
The ultimate goal of clinical decision support systems is to support healthcare professionals by analyzing patient data and offering valuable insights for decision-making in clinical settings. In this article, we delve into how healthcare organizations can utilize CDSSs for this purpose based on successful implementation cases and Mind Studios' own expertise in healthcare software development.
Decision support system development market size
As the pressure on the healthcare system grows due to high patient expectations, the demand for CDSSs in hospitals and other facilities also increases. In fact, according to data from Grand View Research, the global clinical decision support systems market size was valued at $4.9 billion in 2022 and is expected to grow at a compound annual growth rate (CAGR) of 10.6% from 2023 to 2030.
The rising demand for patient-centered, mindful care is one of the key drivers that have triggered the market growth of decision support solutions. However, there are other reasons to be aware of as well, including:
- The immense strain placed on healthcare professionals due to staff shortages and the demand for healthcare services that outpaces the supply of qualified personnel
- Recognition and favorable initiatives from governments worldwide that encourage the adoption of CDSSs and EHR systems within the healthcare systems
- Rising healthcare data volume like electronic health records (EHRs), medical images, and patient-generated health data, all of which CDSSs help leverage for informed decision-making
- The evolution of healthcare platforms in terms of interoperability, AI and ML adoption, telehealth development, etc
- Rapid advancements in biotechnology and bioinformatics that stimulate significant improvements in data storage, management, and analytics processes
- Awareness of the population health management importance, for which CDSS is essential to effectively identify at-risk patients and deliver targeted interventions and preventive care
It’s important to note that clinical decision support systems can be integrated with other healthcare systems like EHR or CPOE. However, the standalone CDSSs held the largest market share of over 31% in 2022 and are expected to dominate the market in the near future due to their low cost, simplicity, and ease of use, which is extremely important in clinical settings.
Benefits of clinical decision support system development in healthcare
Imagine a healthcare system where clinicians are empowered with instant access to all the data they need, from the latest research to patient histories. That’s precisely the function of decision support systems. Ultimately, they are virtual consultants augmenting healthcare professionals' skills. Let’s look closer at the benefits of a decision support system development that healthcare organizations can expect to leverage.
Reduced medical errors and adverse events
The healthcare industry has made a huge leap thanks to digitalization, but the medical error rate is still higher than one might think. For instance, in the US alone, an estimated 1.2 million patients deal with the consequences of medical mistakes made in hospitals.
Medical errors and adverse events can be caused by dozens of factors, from miscommunication to medical workers simply not having enough time due to extremely high workloads. While clinical decision support systems cannot eliminate these events, they contribute significantly to patient safety.
To be more specific, CDSSs review patient data, cross-reference it with clinical guidelines, and provide real-time alerts and recommendations, thus assisting healthcare providers in making informed decisions.
For example, the segment of CDSS that accounted for the largest market share (over 26.0%) in 2022 was drug allergy alerts, which help detect medicine errors during the process of prescribing, dispensing, or administering medications.
Preventive care facilitation
Many conditions that become deadly can be manageable and even preventable when detected early — and that is why preventive care is one of the most vital trends in the healthcare industry. For instance, chronic diseases like heart conditions and diabetes are responsible for 7 out of 10 deaths in the US. Yet, according to the Centers for Disease Control and Prevention, preventive care services could save over 100,000 lives in the country every year.
Preventive screenings, vaccinations, and health maintenance are essential for early disease detection and prevention. However, the high number of patients per healthcare professional and the complexity of patient care make it challenging to ensure timely and comprehensive preventive interventions. That’s where CDSSs come in handy.
Clinical decision support systems can be used to maintain meticulous records of patient histories and send automated reminders for vaccinations, screenings, and health check-ups. As a result, they facilitate a proactive approach to healthcare and ensure that no preventive opportunity is missed, contributing to healthier patient populations.
Improved diagnostic accuracy and treatment planning
The complexity of medical conditions, limited time for evaluation, fragmented patient data, overlapping symptoms, rare conditions, human errors, and resource constraints are just a few factors that make accurate diagnosis challenging for doctors. Clinical decision support systems aim to reduce the impact of these intricacies and assist healthcare professionals in enhancing diagnostic accuracy and treatment planning.
Here is how it works: clinical decision support systems analyze patient data comprehensively, which helps identify potential conditions, suggest relevant medical tests, and offer evidence-based recommendations. This empowers clinicians to make informed decisions and eliminate incorrect treatments, delayed recoveries, or worsening of conditions.
Automated data analysis and reporting
Each year an annual hospital produces roughly 50 petabytes of data, according to HealthTech Magazine.
Continuous accumulation of medical data enables HCPs to identify trends, patterns, and insights essential for evidence-based decision-making in patient care. The challenge here lies in the volume of this information. An average hospital produces roughly 50 petabytes of data annually, so it’s no surprise that the healthcare industry generates around 30% of the world’s data volume.
Without robust data analysis tools, accumulating this data would be pointless. Therefore, decision support systems assist healthcare organizations in data analysis and reporting, sifting through vast datasets and extracting meaningful insights that guide HCPs.
Adherence to clinical protocols and guidelines
Clinical protocols and guidelines are standardized, evidence-based sets of recommendations and procedures that serve as a foundation for consistent and high-quality patient care. They provide a framework for consistent diagnosis, treatment, and patient management, fostering a culture of quality and accountability in healthcare facilities. Therefore, strict adherence to them is essential in clinical settings.
So, what’s the role of decision support systems in this process? DSSs facilitate adherence to clinical protocols and guidelines by providing real-time alerts, evidence-based recommendations, and structured pathways for HCPs. In treatment-related tasks, for instance, they analyze patient data, compare it to established best practices, generate reminders for preventive care, and offer treatment approaches, ensuring that healthcare providers follow up-to-date guidelines.
Increased workforce efficiency
Due to healthcare's demanding and highly stressful nature, every moment in a clinical setting counts. Therefore, decision support systems are used not just for patient care but for streamlining administrative tasks and paperwork to free the valuable time of medical workers.
Healthcare organizations and facilities often use DSSs to automate tasks like appointment scheduling, patient registration and documentation, billing and claims processing, inventory management, and workflow optimization.
The systems streamline these tasks through data automation, intelligent algorithms, and real-time monitoring. For instance, for appointment scheduling, DSS utilizes algorithms to optimize appointment slots based on provider availability and patient preferences. For billing and claims processing, such systems can automatically generate invoices, validate insurance information, and flag potential billing errors.
The biggest value of DSS used for administrative processes is that it allows healthcare workers to dedicate more time to meaningful patient interactions, build trustworthy relationships, and ultimately fulfill their purpose.
Efficient resource utilization and cost-effective care delivery
Another advantage of DSSs that isn’t directly related to the quality of care is cost efficiency. Considering the limited resources of medical facilities, including personnel, equipment, and finances, healthcare executives simply have to maximize those resources to their fullest potential to provide adequate patient care.
Decision support systems can be quite helpful in promoting efficient resource utilization and cost-effective care delivery. Some tasks they are used for include patient data analysis, cost analysis and reporting, streamlining clinical workflows, automating compliance monitoring, identifying cost reduction opportunities, and employing predictive analytics.
All this enables healthcare organizations to allocate resources effectively, reduce unnecessary expenses, and enhance clinical efficiency while maintaining financial stability, ultimately benefiting both patients and the healthcare system.
Challenges of decision support systems in healthcare
As with most technologies, DSSs come with complex challenges that healthcare executives have to address and navigate. In this section, we dive into the critical of those and explore the strategies and solutions available.
Data privacy and security concerns
With the increasing digitization of health records and reliance on DSS, protecting patient data has become one of the top priority tasks for healthcare organizations. Decision support systems rely heavily on vast amounts of sensitive data, which makes them high-risk targets for cyberattacks. Any breach can have severe consequences, including legal repercussions and reputation losses.
However, the most critical consequence would be harming patient safety since medical data breaches can result in people facing identity theft, loss of control over their records, delayed care, and compromised treatment plans.
Healthcare executives must prioritize data privacy and security by investing in robust encryption, access control implementation, continuous monitoring and regular DSS security audits, staff training, and adherence to industry standards like HIPAA. Collaboration with cybersecurity experts and staying informed about emerging threats can also help healthcare organizations protect data security.
User acceptance and usability challenges
No matter the potential advantages of decision support system development, the effectiveness of DSS heavily depends on whether the healthcare professionals are willing to embrace and effectively utilize it. Therefore, user acceptance and usability are pivotal factors in successfully implementing CDSS in healthcare.
HCPs may encounter challenges in adopting and using DSS effectively due to the system’s complexity, inadequate training and support, interoperability challenges, and simply resistance to change when the system's value is not communicated properly.
To address this challenge, it is vital to involve and engage the users in DSS development, design, implementation, and evaluation, as well as provide comprehensive training, support, and feedback to healthcare workers. Additionally, it is important to establish and follow key performance indicators (KPIs) to measure the impact of DSS on patient outcomes, thus highlighting the value of the system.
Data quality and availability
Let’s say a hospital's DSS for drug recommendations was fed data of poor quality. Inconsistent and incomplete medication data in the EHR system compromised the DSS's ability to identify drug interactions and provide appropriate medication suggestions accurately. Furthermore, data accessibility issues hindered the DSS's real-time functionality. As a result, the healthcare professionals' trust in the system has crumbled, and they now bypass it and rely on their own judgment only.
Poor data quality and availability can lead to inaccurate DSS outputs, render the system unreliable, and erode user trust. Therefore, integrating decision support systems into healthcare workflows requires ensuring data quality (its accuracy, consistency, timeliness, and relevance) and availability (its accessibility, security, and reliability).
For this, executives of healthcare organizations must establish data governance programs to maintain data accuracy and implement data validation processes, regular data audits, and data quality standards to ensure that the information used by DSS is reliable. Additionally, it’s crucial to invest in data quality initiatives like staff training and data stewardship roles within the organization is crucial.
Potential biases and limitations of decision support systems
Unfortunately, decision support systems in healthcare are not immune to biases and limitations. After all, they utilize human-generated data, reflecting healthcare system disparities. DSSs can introduce biases into their recommendations, which primarily come from three sources: patient demographics, healthcare provider behavior, and historical data.
Let’s say a DSS for predicting post-discharge readmission risks relies on historical patient data, which contains a bias toward overrepresenting patients from higher socio-economic backgrounds with lower readmission rates. The DSS then underestimates readmission risks for patients from disadvantaged socio-economic backgrounds, potentially leading to inadequate post-discharge care.
Recognizing and addressing such biases is essential to ensure that DSS recommendations are equitable and can positively impact healthcare outcomes. For this, healthcare providers should work closely with data scientists, continuously monitor algorithms, conduct regular audits, and train the system on more diverse databases.
Integration with existing healthcare systems and interoperability issues
Healthcare facilities typically have multiple established solutions, such as EHR systems, that store and manage patient data. Integrating a DSS with these existing systems can be challenging, especially when the existing solutions haven’t been updated for a while. As a result, DSSs might be unable to communicate with and retrieve data from the established systems without disrupting their regular operation.
The technical and operational challenges associated with DSS integration is typically caused by incompatibility of data formats, protocols, standards, and interfaces, and security, maintenance, and coordination issues. To eliminate these challenges, healthcare organizations must adopt and follow best practices, methods, and tools to facilitate system integration and interoperability.
For instance, healthcare executives can adopt interoperability initiatives and standards like FHIR® (Fast Healthcare Interoperability Resources), encourage data sharing, and foster a culture of interoperability to break down silos and improve data access.
Real-world examples of successful decision support systems
One of the earliest clinical decision support system development projects, MYCIN, emerged in the 1970s. Back then, this rules-based DSS required physicians to manually enter patient data (which took over 30 minutes) so that the system could run it against around 600 rules to diagnose infectious diseases and suggest antibiotics. However, MYCIN was primarily used for research at Stanford Medical School and didn’t get adopted in clinical settings.
Today, clinical decision support systems are rapidly making their way into healthcare systems worldwide. Knowledge-based and non-knowledge-based, ML-powered, predictive, and mobile — CDSSs encompass various types tailored to specific healthcare needs. Let’s look at some of the most successful clinical decision support systems cases that made it into real-world healthcare environments.
PathAI
PathAI is a provider of AI-powered clinical decision support tools that assist pathologists in making accurate diagnoses for each patient, advancing precision medicine.
After scanning and digitizing pathology slides, the PathAI system’s advanced machine-learning algorithms analyze these slides and detect even subtle signs of cancer cells or abnormalities that the human eye would likely have missed. As a result, pathologists can explore the highlighted regions of interest and use these findings for timely cancer diagnosis, thus increasing the chances of successful treatment.
Having gained $355.2 million in funding since 2016, PathAI has partnered with multiple healthcare facilities. In 2022, the company announced a 5-year strategic collaboration with Cleveland Clinic, an academic medical center encompassing 19 hospitals and more than 220 outpatient clinics. Thanks to this partnership, PathAI gained access to de-identified digitized pathology slides and clinical data to enhance its AI algorithms for research and validation.
Philips HealthSuite Imaging
Developed by Philips Healthcare, the HealthSuite Imaging platform-as-a-service (PaaS) solution aims to advance medical imaging and diagnostic processes by integrating a wide range of healthcare data, imaging modalities, and clinical applications.
In spring 2023, Philips announced that HealthSuite will use Amazon HealthLake Imaging. This collaboration aims to expand the platform's scale, speed up the process of accessing initial medical images, facilitate the reuse of images for ML applications and research, and reduce medical imaging costs.
By developing solutions on Philips HealthSuite powered by AWS (Amazon Web Services), Philips also aims to enable faster patient care and substantially reduce human errors. The company’s goal is to positively impact 2.5 billion lives annually by 2030.
Last but not least, Philips expects that introducing the imaging solution of AWS will help enhance data management for radiologists and clinicians throughout the imaging workflow, from diagnosis to follow-up. Using the cloud solution will also enable HCPs to access innovations remotely, reducing on-site hardware and data center expenses.
Zynx Health
Zynx Health, a part of the Hearst Health network, is the market leader in providing evidence-based and experience-based clinical decision support solutions that enhance patient care quality, safety, and efficiency.
The company has already gained trust of thousands of hospital organizations and providers that want to drive clinical improvements and make meaningful use of EHR systems. Collectively Zynx Health clients have customized and deployed over 100,000 order sets and care plans within the company’s online solutions.
One of the Zynx Health knowledge management solutions, Knowledge Analyzer, assists hospitals in aligning clinical decision support tools effectively and prioritizing interventions for the greatest positive impact on patient outcomes. It also streamlines the evaluation of order sets, care plans, protocols, rules, policies, procedures, and other content so that HCPs can concentrate on improving and standardizing patient care.
In 2023, Zynx Health was ranked #1 in KLAS Research’s 2023 Best in KLAS Awards in the category of Clinical Decision Support — Care Plans and Order Sets. Moreover, this event marked the third consecutive year in which Zynx Health has been awarded top honors by KLAS Research.
Mind Studios as your custom healthcare software development partner
Healthcare is a challenging field to develop software solutions for. Mind Studios knows this from experience.
Over the years, our development team built multiple products for the healthcare industry. These include a thriving mHealth app for people with chronic illnesses, a medical knowledge base platform, and a solution for a medical startup that our client, a board-certified dermatologist, successfully exited after years of profiting from it.
The most important lesson we learned from our healthcare projects is that to succeed, medical software has to go beyond advanced technological solutions. As software experts, we must develop not just the platform but a long-term strategy to ensure the solution benefits the healthcare organization in question.
Working closely with healthcare professionals, we get their concerns: custom software development can be costly, so the investment has to be worth it in the long run. Therefore, we start by meticulously studying the organization’s operations and the needs of the intended users. This helps us develop solutions that help reduce administrative costs, streamline clinical workflows, support the organization in its transition to digital data management, and protect it from data security breaches (which can also be expensive if it comes to legal repercussions).
Naturally, with the development of a decision support system, we take the same approach. Our fundamental goal in this task is to take away at least part of the burden healthcare professionals deal with and empower them with easy-to-use, intuitive guidance systems. The tech solutions are just our tools to achieve this goal.
Conclusion
Even the most knowledgeable practitioners require a supportive environment and assistance with quick access to reliable and relevant information to do their job with surgical precision. With a meticulous development approach, clinical decision support systems can become healthcare providers’ trusted navigators, helping them leverage the enormous amounts of medical data and serve patients more effectively.
As an experienced healthcare software development company, Mind Studios can help your organization implement a clinical decision support system, which will become part of the powerful synergy between medical workers and technology.
Feel free to request a free consultation so that our business development team can guide you through the optimal CDSS development and implementation strategy aimed at enhancing the quality of healthcare you deliver.