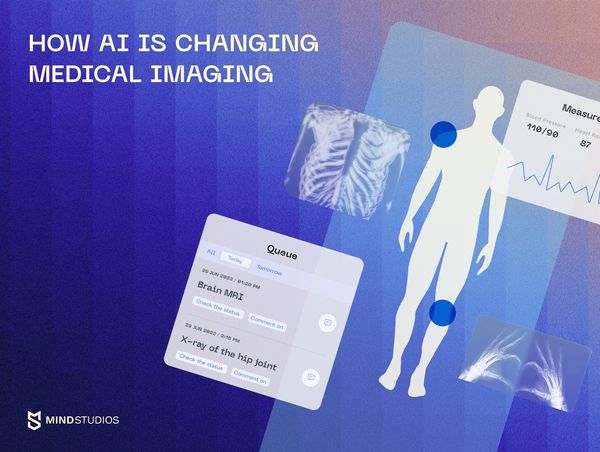
Due to its potential to revolutionize healthcare systems, the concept of using artificial intelligence (AI) in medical practice has attracted significant attention. However, the unpredictability of AI systems and the never-ending list of ethical and legal concerns made it so only a few AI algorithms have been implemented so far.
One particularly useful use of artificial intelligence is in the realm of content creation, where generative AI excels. And there is content to be created in the medical field. Medical imaging AI has numerous uses in medicine and related fields from better diagnostic imaging analysis to individualized care. That said, there are several other ways in which healthcare stakeholders might benefit from generative AI.
The value of AI in the healthcare industry was US$15.4 billion in 2022, and Grand View Research predicts it to grow at a CAGR of 37.5% from 2023 to 2030. As a result, generative AI and other forms of artificial intelligence will become commonplace in the medical field.
The recent surge in the development of AI technology and the benefits it may bring to the healthcare business have been the main focus of our interest at Mind Studios, where we have been producing specialized healthcare solutions for years. In this article, we'll go over how medical imaging AI can help fix some of the healthcare system's biggest problems.
What is the role of AI in medical imaging and how is it used?
AI and medical imaging assists healthcare workers in identifying trouble regions or details that the human eye may overlook. AI-powered medical imaging, for example, may evaluate data points in a medical report to differentiate a disease (from a healthy portion) and signals (from noise). AI-powered medical imaging is often used to:
- Recognize complicated patterns in image data
- Provide a quantitative assessment of radiographic characteristics
- Identify picture modalities at various stages of therapy (for example, tumor delineation)
- Discover illness features that are not visible to the naked eye
Now developers and radiologists are cooperating to create and train algorithms that are accurate enough for clinical use. In the US, every AI algorithm requires the approval of the U.S. Food and Drug Administration (FDA) before implementation. By 2022, FDA has approved 521 AI solutions, 75% of which belong to radiology, a field that requires extreme accuracy. Actually, some AI-powered software solutions in radiology already boast over 90% accuracy, which got our team's attention.
AI techniques used in medical imaging
Among all the AI techniques, the most commonly used in the medical imaging industry are machine learning, deep learning, and computer vision. Let’s take a look at what exactly each of them brings to radiology.
Machine Learning
Machine learning is one of the technologies for artificial intelligence, and it aims to imitate the process of human learning with the use of algorithms and data to train from. In medical imaging, machine learning is used in training the AI on medical scans and other images to grant it the ability of pattern recognition. With this ability, the model becomes capable of making predictions and classifications of diagnoses based on the provided image.
Deep Learning
Deep learning is a subset of machine learning, representing a neural network able to simulate brain behavior. This ability grants it to also simulate learning processes from large volumes of data. A neural network usually consists of no less than three layers, and with only one layer it can already be used for predictions. The other layers are used to improve the accuracy and thoroughness of such predictions, making the neural network more optimized. The deep learning technique in medical imaging is expected to assist radiologists in providing more accurate diagnoses and analyzing suspicious lesions.
Computer Vision
Computer vision is another technology for artificial intelligence that makes it capable of processing both 2D and 3D images, videos, and models. In medical imaging, computer vision enables the AI to recognize and identify known issues in the provided medical scan, as well as analyze and differentiate between them.
Benefits and challenges of AI in medical imaging
As artificial intelligence is growing in abilities rapidly, it’s easy to predict its popularity in medical imaging in the nearest future. However, it’s important to understand the benefits of its adoption right now and not overlook the challenges it brings. To sum up everything we've mentioned above, we believe the most important benefits of medical imaging AI are:
- More accurate classifications. Enhanced with deep learning and computer vision algorithms, artificial intelligence is able to recognize even the smallest abnormalities in the provided scans regardless of their location. With greater pattern recognition abilities and large volumes of training data, AI can accurately classify the lesion it identifies.
- Enhanced analysis. With proper training, AI models are capable of providing detailed information about the recognized lesions, usually serving as a second opinion for radiologists in practice or leading to a point hidden from a naked eye of a specialist.
- Creation of 3D models. Artificial intelligence is not only able to analyze 3D models thanks to computer vision but can also assist in their creation. For a more thorough medical imaging analysis, AI can 3D visualize a part of a patient’s body to provide better diagnostics. For example, there is an AI that 3D models the coronary arteries of a patient based on their coronary CT scans.
- Faster diagnosis. Human doctors take time to carefully read the imaging, it's part of the diagnosing process. For example, in cardiology, radiologists spend around 13 minutes diagnosing a patient, and with AI, they are able to do it in 20 seconds.
When it comes to the challenges of AI in medical imaging, the crucial one is definitely privacy issues. To train a model properly, medical researchers need to access an existing dataset from patients’ medical and personal records. This becomes a big stumbling block for institutions, as there are many patients who are against participating in a model’s training with their personal data, and have the right to do so.
The first task for technology implementation is to gain the trust of the patients. As for now, AI technologies are not even HIPAA compliant, so the lack of trust in technology is quite justifiable.
As artificial technology is a relatively new trend for medical imaging, there are yet no standards for its implementation. This leads to a trial-and-error method, which significantly slows the process of AI adoption. As not many companies are willing to risk their resources for this technology right away, some choose to keep their hand on the pulse but not directly involve themselves yet.
Modern challenges of medical imaging and AI for healthcare data visualization
The primary issues that the healthcare industry faces may be summed up in two words: high costs and poor patient experiences. However, there are several technical obstacles that occur when implementing and employing AI for medical imaging.
Medical imaging
Medical images in the majority of imaging modalities generally suffer from one or more of the following problems during acquisition:
- Low resolution (both spatial and spectral)
- High noise levels
- Lack of contrast
- Geometric deformations
- Imaging artifacts
The introduction of fresh methods in the medical field, such as newer scanners that give new imaging modalities, poses difficulties to medical data visualization research — human professionals spend a lot of time on processing all that data. Besides, in a medical environment, different data kinds are accessible, such as medical imaging scanners, sensors, and patient information. AI can process this huge load of old and new data faster than a human ever could.
Healthcare data visualization
Because of noise, confounding effects, and a lack of standardization in metadata capture, these data types may be unclear, noisy, varied, and difficult to interpret. The core issues of medical data visualization, however, are usually the following:
- Data preparation, which is crucial for high-quality visualization, includes such time-consuming tasks as picture enhancement, segmentation, and data transformation.
- Lack of collaboration between image processing researchers and the development of a taxonomy of medical activities. When done right, it can make medical graphics studies easier to understand and more useful in everyday life.
- Extracting features from medical data is challenging and often requires expert decision-making.
- Data assimilation, combining observed data with computer models, can improve accuracy but faces difficulties in data availability, curation, and ethical issues.
- Access to freely available data is important for innovative visualization approaches, but legal restrictions and patient permission often limit its availability. Likewise, ethical concerns and the lack of regulation standards contribute to challenges in data privacy and usage.
- Standardization is a problem in medical visualization due to the lack of established standards and harmonization for modern imaging methods.
The creation of cutting-edge methods in the healthcare sector is a major motivator for researchers working in the field of medical visualization despite the need for medical licensing. For instance, the introduction of innovative scanners results in novel imaging modalities, each of which has its own set of visual problems. We keep an eye on healthcare technology breakthroughs, such as AI solutions, at Mind Studios and can incorporate them into an existing model or design one for you. Because healthcare is one of our expertise, we know how to create a product that stands out.
How does AI help solve these issues?
The medical industry makes use of a wide range of data types, from patient records to medical imaging scanner and sensor data. One scanner may collect several different types of information, such as multivalued data, as well as single scalar, tensor, and vector fields. Unstructured, partial, or inconsistent medical data might make analysis challenging. This might be due to a number of things, such as inconsistent metadata capture or data noise. X-ray exposure reduction and patient movement are two more possible explanations.
Artificial intelligence can improve several aspects of diagnostic and interventional radiology, including image processing, diagnosis, intervention prescription, clinical predictive modeling, and education.
Better diagnosis efficiency with generative AI in medical imaging
Human error in healthcare settings may be more common due to high patient volumes and a lack of relevant medical history. Artificial intelligence technologies can detect and diagnose illnesses quicker than human doctors while offering better interpretability of medical images.
Reducing healthcare costs
When generative AI in healthcare visualization is used to streamline the diagnostic process, it helps keep healthcare costs down. Imagine a scenario in which AI can search through millions of diagnostic photos for signs of sickness. Manual work is rendered unnecessary. The requirement for inpatient stays can be minimized, as can the time spent waiting for treatment.
Simplifies information sharing
The promise of AI in precision medicine relies on the speed and accuracy with which its algorithms can process large datasets. For instance, in the United States, diabetes now affects 11.3% of the population. Urgent treatment and management are required, and AI can aid medical personnel in making sense of data from a continuous glucose monitoring device to better comprehend the disease.
Applications of AI in medical imaging
Today, there is no actual AI yet that can completely replace a radiologist, but is there a way of implementing AI to enhance the existing processes? In this section, we are going to talk about practical applications for artificial intelligence in medical imaging, and how it can play the role of a “second reader”.
Brain tumor
Traditionally, classifying a brain tumor means surgery, where a doctor removes as much of the tumor’s mass as possible to analyze the sample thoroughly. It takes time for the sample to be processed, stained, and delivered to a pathologist for analysis. Although AI is incapable of replacing the operation stage, it can enhance the sample analysis and save some precious time for the patient.
For example – DeepGlioma AI was able to classify the tumors of 153 patients into correct subgroups with an accuracy of 93.3%. DeepGlioma neural network algorithms analyze brain tumor tissue scans and classify them based on the World Health Organization (WHO) classification system. Along with great accuracy, this AI imaging solution also provides results in under 90 seconds.
Breast cancer
Recent researches show that machine-learning models can only slightly assist doctors in breast cancer screening and hence are still far from widespread implementation.
For example, a trained AI model was able to increase the diagnostics accuracy for ultrasound examinations of breast cancer. In a study, 10 different radiologists diagnosed 663 breast exams without AI’s help and had an average accuracy of 92%. Then, the assistance of the AI tool for medical imaging helped to increase the overall accuracy in those examinations to 96%.
However, for a more advanced type of screening — mammography, the AI showed worse accuracy than the decisions of practicing professionals. A combination of three studies involving more than 79,900 screened women compared 36 AI tools with the clinical decisions of radiologists. 34 out of 36 solutions were less accurate than a decision of a single specialist, and every single one of them performed worse than the consensual decision of two radiologists.
These studies lead to the conclusion that it’s too early to adopt artificial intelligence for clinical use in an advanced type of screening, but for more common ones it can already be of assistance.
Neurological diseases
As the main source of medical imaging in detecting neurological diseases is MRI scans of the brain, it takes time to analyze the results and find any vital changes that signal a developing condition. Unfortunately, human sight is imperfect, and when it comes to minor differences, even an experienced specialist may fail to notice them. Artificial intelligence can add some precision to classifying these scans, leaving fewer signals unnoticed.
To study the impact of AI-powered tools in neurological screening, the researchers allocated 656 brain features across the 115 regions of a brain that flag pathologies related to Alzheimer’s disease (AD). Trained on this segmentation, the machine-learning solution required only one brain scan on an MRI 1.5 Tesla model, to identify and predict the development of AD at early stages. The core algorithms of the model are already-known ones for classifying cancer tumors, adapted for working with a brain only.
Eventually, this model, trained on MRI scans from the Alzheimer’s Disease Neuroimaging Initiative, was able to correctly differentiate brain scans of patients with AD in 98% of cases.
Cardiovascular issues
Artificial intelligence can be useful in detecting heart diseases on cardiovascular MRI scans. Research shows that a machine learning algorithm trained on 1923 CMR scans from 13 different institutions was used in segmenting left ventricular blood volume and myocardium. Not only the fact of usage claims our attention, but also the statistics, showing only 1 in 479 cases of mis-segmentation.
This great precision of the algorithm also takes about 20 seconds per decision, greatly surpassing manual diagnostics, which take around 13 minutes on average. This shows that with greater training and a bigger number of scans to train from, AI can be both efficient and time-saving in detecting cardiovascular issues early.
Radiation dosage reduction
Medical imaging by means of MRI or CT inevitably leads to patients being exposed to radiation. As every exposure comes with a harmful effect on the human body, the ways to reduce it are always a challenging topic. Especially when it comes to pediatrics, reducing the dose of radiation in medical imaging can become vital for a growing body. And artificial intelligence has something to offer here.
The solution lies in AI’s deep learning image reconstruction (DLIR). The main advantage of using DLIR in radiology is that it can reconstruct images from lower-dose computed tomography. The main challenge is whether the image is readable enough when reducing the radiation doses and applying the image reconstruction.
And according to a study, convolutional neural networks (CNN) — the most common AI technique and architecture — can reduce radiation dosage by 36–70% without losing any diagnostic information. This study shows that artificial intelligence can play a crucial role in radiation dosage reduction, however, it still requires more thorough research, as only 3 possible imaging modalities (CT, PET/MRI, and mobile radiography) were covered.
Musculoskeletal injuries
As X-rays and CT are still the most common ways of finding any fractures and injuries, they leave space for human errors even when looked at with the eyes of a professional. Artificial intelligence can’t boast a much greater accuracy, as it also makes mistakes, however, it can aid a specialist in diagnosing more precisely and in a shorter amount of time.
In a recent study, an AI-powered tool trained on more than 55,000 scans showed a sensitivity of 92% when detecting fractures. Compared to the sensitivity of 91% that clinicians managed to demonstrate in that study, it is safe to say that AI is coming close to serving as a diagnostic assistant for musculoskeletal injuries.
Use cases of generative AI in areas of healthcare
By improving the analysis of healthcare data and spotting patterns and trends, generative AI is predicted to completely change the healthcare industry. Better machine learning algorithms will increase the scope of applications for generative AI in healthcare, leading to more precise diagnoses and better treatment strategies.
Personalized patient care will be made possible by the combination of generative AI with other technologies, such as medical imaging and wearable health equipment. A more unified and individualized strategy for analyzing and implementing healthcare data is anticipated as a collaboration between providers, academics, and AI technology improves. So, let's take an in-depth look at how generative AI imaging is already assisting doctors and other medical professionals.
Generating high-quality medical images
GANs (generative adversarial networks) are capable of producing high-resolution medical pictures in high detail. Generative AI may be used to produce high-resolution medical pictures, assisting in image quality and detail enhancement. This is very beneficial in fields like radiology and pathology. This is where crisp and precise imaging is critical for correct diagnosis.
A work published in Nature Communications, for example, revealed the use of GANs to create high-resolution brain MRI pictures, which helped increase brain tumor segmentation accuracy.
Aiding in the diagnosis of radiography and pathology
Radiologists and pathologists can benefit from generative AI models when interpreting medical pictures. Indeed, by utilizing deep learning techniques, these models can flag possible anomalies or aid in the identification of certain traits. Furthermore, this contributes to more accurate and efficient diagnostics.
Deep learning, for example, is used in an AI-based image evaluation system created by Google and Northwestern University Feinberg School of Medicine to detect cancerous lung nodules on CT images. While human radiologists often check many 2D lung scans, this approach examines lungs in a single large 3D image, increasing the accuracy of the screening output.
Furthermore, the system has been taught to compare both main and past CT scans, which aids in the prediction of lung cancer malignancy chances. It examines both the area of interest and those with a high risk of lung cancer. When both main and previous CT scans are accessible, the method performs similarly to experienced radiologists, and it exceeds them when a prior scan is not available.
Convolutional neural networks (CNNs) and other generative AI models, on the other hand, can help radiologists and pathologists diagnose illnesses. According to research published in Lancet Oncology, an AI imaging model surpassed human radiologists in identifying breast cancer in mammograms.
Using imaging data to predict disease progression
Longitudinal medical imaging data may be analyzed using generative AI algorithms to forecast illness development. For example, research published in Pubmed employed GANs to accurately forecast Alzheimer's disease development using brain MRI data. So, in the future, these models can give insights into illness trajectories by identifying small changes in pictures over time, as well as assist healthcare providers in making educated decisions about treatment plans and treatments.
Drug discovery
Drug discovery typically takes between three and six years and costs between $600 million to $1.8 billion. In contrast to more conventional approaches, generative AI can assist researchers come up with innovative therapeutic ideas that meet their specific requirements and limits.
It can produce new candidates with comparable features but different structures by training on data relating to the chemical properties of known medications, which might lead to safer and more effective treatments. By evaluating massive amounts of data on drug-target interactions, it can also foretell the efficacy and safety of novel drug candidates.
By evaluating patterns in clinical data, generative AI finds subgroups of patients who are more likely to respond to medicine, hence facilitating personalized drug therapy and better patient outcomes.
Personalized care planning
Personalized treatment planning seeks to personalize healthcare approaches to the unique features of each patient. For this purpose, generative AI systems can be used to enable accurate and optimal treatment options. Furthermore, it is possible to develop treatment regimens for individual patients based on medical history and genetics.
Patient data may be analyzed using generative AI models. In reality, it takes into account medical history, genetic information, and other pertinent data to generate tailored treatment programs. This also includes individual differences and optimizes treatment options, resulting in more effective and focused healthcare treatments.
Best AI-based medical imaging software
Let’s take a more practical look at the already existing AI medical imaging companies and the services they offer. In this section, we will tell you about interesting solutions that are changing medical imaging right now.
Volpara Health
Volpara Health is an AI-based medical imaging solution for early diagnosis of breast cancer. More than 16.5 million patients have assessed their breast density and fibroglandular tissue thanks to Volpara. Powered by machine learning, Volpara provides their customers with 4 useful algorithms:
- TruDensity. Based on the provided mammogram scans, Volpara assesses the volumetric breast density (VBD%) in a typical range from 2 to 35%. This precise number helps radiologists to process the sensitivity of the mammogram and optimize the screening, considering the breast density and its difference.
- TruPGMI. This is an image evaluation algorithm that makes sure of the correct positioning during the imaging so that all of the breast tissue is scanned. This algorithm categorizes positioning as Perfect (P), Good (G), Moderate (M), or Inadequate (I) to create an overall positioning assessment.
- TruRadDose. Instead of sticking to the equipment manufacturer's recommendations, it helps analyze the required dose of radiation based on the patient’s breast density. Thus, it helps avoid excessive radiation use in cases when a lower dosage is applicable effectively.
- TruPressure. This algorithm measures the compression pressure that is applied to the breast to help technologists form a better understanding of the patient experience and estimate the effectiveness of particular mammograms.
With a patient-oriented approach, Volpara became a popular software for medical imaging with more than 400 peer-reviewed publications mentioning the solution.
Qure AI
Qure AI is an example of AI with a deep learning algorithm that helps clinicians with diagnoses and patients with personalized treatment. Helping more than 10 million people around 70 countries, the solution offers various AI-based services with algorithms trained on 8+ million scans. Let’s take a closer look at each of them:
- qXR. AI for chest X-rays. A solution trained on over 4.2 million X-rays helps accurately find and classify lung, bones, heart, and diaphragm lesions in less than a minute. Such quick responses derive from the algorithm that initially separates normal X-rays from suspicious ones not to waste time reporting healthy scans.
- qER. AI for head CTs. qER is able to identify 11 various critical cases on non-contrast head CT and immediately alert the neurocritical care department about it. The tool recognizes fractures, infarcts, and abnormalities, highlights their spots on an overlay, and delivers them to specialists for timely care.
- qCT. AI for lung nodules. The qCT algorithm trained on more than 200,000 chest CTs can identify, monitor and characterize lung nodules found on provided scans. Along with being capable of distinguishing lung lesions from complex anatomical structures on lung CTs, this solution helps clinicians identify lung cancer at its early stages.
- qVH. AI for the ultrasound. This algorithm provides patients with enhanced care for cardiovascular diseases. Automated detection of carotid artery stenosis, and atherosclerotic plaques along with analyzing the potential risks for asymptomatic patients makes it efficient in terms of timely care and early discovery.
- qMSK. AI for musculoskeletal X-rays. The qMSK algorithm is similar in pattern recognition to the qXR one, but the area of specialization differs significantly. This solution is also capable of detecting and classifying issues in less than a minute, however, it aims at a greater area than the chest only. Bone fractures and joint dislocations across the whole skeleton are now easily detected and identified.
The process of bringing AI into medical imaging and healthcare data visualization
In modern medicine, imaging is indispensable. The inner workings of the human body may be examined in great depth. The cost, accuracy, and patient safety of modern medical technologies are constantly evolving.
Recent advances in artificial intelligence, from machine learning to deep learning and generative AI models, have shown potential methods for addressing a wide range of intractable issues across a number of sectors. Following is some advice in case you plan to implement generative AI models in your healthcare business.
Talk to medical professionals and experts
The use of AI in healthcare has to be promoted by doctors and other stakeholders. They may give useful input on the AI system's design and functioning if they're brought in early on in the process. This allows you to determine whether the AI is suitable for the hospital and address any issues that may arise.
Prioritize data integrity and safety
Since healthcare AI relies heavily on data, ensuring its quality and safety is paramount. Data storage and pre-processing to eliminate mistakes and inconsistencies are also part of this. Protected patient data-sharing systems need to be developed.
Pick an AI technology
Generative AI and natural language processing (NLP) are two of the primary areas where AI might be useful in healthcare. It's crucial to choose the appropriate technology for your business and tasks. In order to assess your options and make an informed decision, you may choose to hire a consultant or an AI development company.
Closely monitor and evaluate the therapy done with AI
To ensure the success of medical imaging AI in healthcare, it must be constantly evaluated. This may entail monitoring crucial performance metrics and the AI system frequently to detect issues and opportunities for improvement. Businesses need to remain flexible to continue benefiting from AI systems.
Double-check the AI model
Artificial intelligence in medical imaging has to be developed and tested first. Simulations and small-scale experiments are useful for this purpose. It is the responsibility of clinicians and other stakeholders to assure the efficacy and reliability of AI.
At this stage, pattern or data analytics may guide therapeutic recommendations, or AI may automate patient care. To evaluate the answer, you must understand the issue and its success.
Focus on high-grade data security
Because AI in healthcare relies on data, quality and security are essential. This may include cleaning and pre-processing data to eliminate mistakes and inconsistencies and securely storing data according to standards. Organizations must also build data sharing mechanisms that preserve patient privacy.
Don’t forget about medical imaging AI testing and validation
After design, the AI system should be tested and verified before clinical use. Simulations or pilots can identify technological problems and improvement areas. Clinicians and other stakeholders must be engaged to ensure AI effectiveness and reliability.
Provide training and assistance
Finally, clinicians and personnel require training and help to effectively use generative AI for medical imaging data gathering and visualization. This may involve technological training as well as any new processes or workflows. All employees must be familiar with the AI system, understand it, and be able to communicate how it may improve patient care.
Conclusion
Armed with machine learning, deep learning, and computer vision, AI technologies are knocking on the door to aid radiologists and save time for their patients. AI has already found its applications in the early detection and classification of brain tumors, breast cancer, neurological diseases, cardiovascular issues, and musculoskeletal injuries based on the provided MRI, CT, and X-ray scans.
Despite being quite challenging, there are significant benefits to adopting AI for medical imaging. Quicker diagnosis, enhanced analysis, and deeper lesion classification are heavy arguments for implementing artificial intelligence into your business.
We at Mind Studios enjoy helping our customers with unique healthcare solutions and will be happy to help you pick the right AI solution for your exact business needs. In case you have any questions or a great idea in mind – feel free to contact us, and our analysts will reach out to you shortly.